Open Access
ARTICLE
ER-Net: Efficient Recalibration Network for Multi-View Multi-Person 3D Pose Estimation
1 National and Local Joint Engineering Laboratory of Computer Aided Design, School of Software Engineering, Dalian University, Dalian, 116622, China
2 School of Computer Science and Technology, Dalian University of Technology, Dalian, 116024, China
* Corresponding Authors: Rui Liu. Email: ; Dongsheng Zhou. Email:
(This article belongs to the Special Issue: Recent Advances in Virtual Reality)
Computer Modeling in Engineering & Sciences 2023, 136(2), 2093-2109. https://doi.org/10.32604/cmes.2023.024189
Received 30 May 2022; Accepted 21 September 2022; Issue published 06 February 2023
Abstract
Multi-view multi-person 3D human pose estimation is a hot topic in the field of human pose estimation due to its wide range of application scenarios. With the introduction of end-to-end direct regression methods, the field has entered a new stage of development. However, the regression results of joints that are more heavily influenced by external factors are not accurate enough even for the optimal method. In this paper, we propose an effective feature recalibration module based on the channel attention mechanism and a relative optimal calibration strategy, which is applied to the multi-view multi-person 3D human pose estimation task to achieve improved detection accuracy for joints that are more severely affected by external factors. Specifically, it achieves relative optimal weight adjustment of joint feature information through the recalibration module and strategy, which enables the model to learn the dependencies between joints and the dependencies between people and their corresponding joints. We call this method as the Efficient Recalibration Network (ER-Net). Finally, experiments were conducted on two benchmark datasets for this task, Campus and Shelf, in which the PCP reached 97.3% and 98.3%, respectively.Graphic Abstract
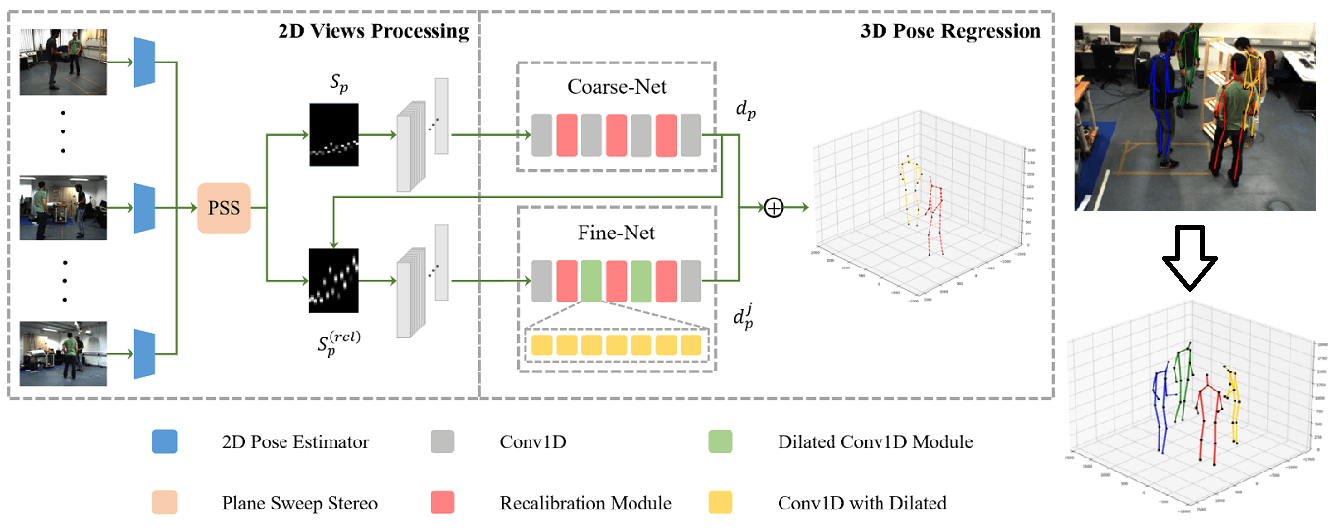
Keywords
Cite This Article
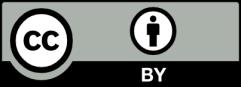