Open Access
ARTICLE
TC-Fuse: A Transformers Fusing CNNs Network for Medical Image Segmentation
1
School of Information Sciences and Technology, Shijiazhuang Tiedao University, Shijiazhuang, 050043, China
2
College of Resources and Environment, Xingtai University, Xingtai, 054001, China
3
School of Electrical and Electronic Engineering, Shijiazhuang Tiedao University, Shijiazhuang, 050043, China
* Corresponding Author: Ying Zhang. Email:
(This article belongs to the Special Issue: Computer Modeling of Artificial Intelligence and Medical Imaging)
Computer Modeling in Engineering & Sciences 2023, 137(2), 2001-2023. https://doi.org/10.32604/cmes.2023.027127
Received 17 October 2022; Accepted 02 February 2023; Issue published 26 June 2023
Abstract
In medical image segmentation task, convolutional neural networks (CNNs) are difficult to capture long-range dependencies, but transformers can model the long-range dependencies effectively. However, transformers have a flexible structure and seldom assume the structural bias of input data, so it is difficult for transformers to learn positional encoding of the medical images when using fewer images for training. To solve these problems, a dual branch structure is proposed. In one branch, Mix-Feed-Forward Network (Mix-FFN) and axial attention are adopted to capture long-range dependencies and keep the translation invariance of the model. Mix-FFN whose depth-wise convolutions can provide position information is better than ordinary positional encoding. In the other branch, traditional convolutional neural networks (CNNs) are used to extract different features of fewer medical images. In addition, the attention fusion module BiFusion is used to effectively integrate the information from the CNN branch and Transformer branch, and the fused features can effectively capture the global and local context of the current spatial resolution. On the public standard datasets Gland Segmentation (GlaS), Colorectal adenocarcinoma gland (CRAG) and COVID-19 CT Images Segmentation, the F1-score, Intersection over Union (IoU) and parameters of the proposed TC-Fuse are superior to those by Axial Attention U-Net, U-Net, Medical Transformer and other methods. And F1-score increased respectively by 2.99%, 3.42% and 3.95% compared with Medical Transformer.Keywords
Cite This Article
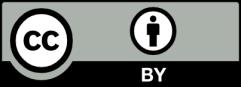