Open Access
ARTICLE
Building Indoor Dangerous Behavior Recognition Based on LSTM-GCN with Attention Mechanism
1
School of Management, Shenyang Jianzhu University, Shenyang, 110168, China
2
School of Information and Communication Engineering, North University of China, Taiyuan, 030051, China
3
School of Information and Control Engineering, Shenyang Jianzhu University, Shenyang, 110168, China
* Corresponding Author: Zhijun Gao. Email:
(This article belongs to the Special Issue: Advanced Intelligent Decision and Intelligent Control with Applications in Smart City)
Computer Modeling in Engineering & Sciences 2023, 137(2), 1773-1788. https://doi.org/10.32604/cmes.2023.027500
Received 01 November 2022; Accepted 13 February 2023; Issue published 26 June 2023
Abstract
Building indoor dangerous behavior recognition is a specific application in the field of abnormal human recognition. A human dangerous behavior recognition method based on LSTM-GCN with attention mechanism (GLA) model was proposed aiming at the problem that the existing human skeleton-based action recognition methods cannot fully extract the temporal and spatial features. The network connects GCN and LSTM network in series, and inputs the skeleton sequence extracted by GCN that contains spatial information into the LSTM layer for time sequence feature extraction, which fully excavates the temporal and spatial features of the skeleton sequence. Finally, an attention layer is designed to enhance the features of key bone points, and Softmax is used to classify and identify dangerous behaviors. The dangerous behavior datasets are derived from NTU-RGB+D and Kinetics data sets. Experimental results show that the proposed method can effectively identify some dangerous behaviors in the building, and its accuracy is higher than those of other similar methods.Keywords
Cite This Article
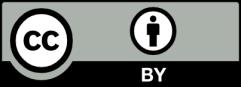