Open Access
ARTICLE
Broad Federated Meta-Learning of Damaged Objects in Aerial Videos
1
School of Aerospace Engineering, Xi’an Jiaotong University, Xi’an, 710049, China
2
Research Institute of Intelligent Engineering and Data Applications, Shanghai Institute of Technology, Shanghai, 201418, China
3
Research Center for Ecology and Environment of Central Asia, Chinese Academy of Sciences, Urumqi, 830011, China
4
Applied Nonlinear Science Lab, Anand International College of Engineering, Jaipur, 391320, India
5
Department of Visual Engineering, International Academy of Visual Art and Engineering, London, CR2 6EQ, UK
6
Institute of Intelligent Management and Technology, Sino-Indian Joint Research Center of AI and Robotics, Bhubaneswar,
752054, India
* Corresponding Author: Wenfeng Wang. Email:
(This article belongs to the Special Issue: Federated Learning Algorithms, Approaches, and Systems for Internet of Things)
Computer Modeling in Engineering & Sciences 2023, 137(3), 2881-2899. https://doi.org/10.32604/cmes.2023.028670
Received 31 December 2022; Accepted 21 March 2023; Issue published 03 August 2023
Abstract
We advanced an emerging federated learning technology in city intelligentization for tackling a real challenge— to learn damaged objects in aerial videos. A meta-learning system was integrated with the fuzzy broad learning system to further develop the theory of federated learning. Both the mixed picture set of aerial video segmentation and the 3D-reconstructed mixed-reality data were employed in the performance of the broad federated meta-learning system. The study results indicated that the object classification accuracy is up to 90% and the average time cost in damage detection is only 0.277 s. Consequently, the broad federated meta-learning system is efficient and effective in detecting damaged objects in aerial videos.Keywords
Cite This Article
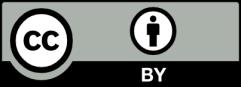