Open Access
ARTICLE
A Degradation Type Adaptive and Deep CNN-Based Image Classification Model for Degraded Images
School of Information Technology and Management, Hunan University of Finance and Economics, Changsha, 410205, China
* Corresponding Author: Wei Wang. Email:
(This article belongs to the Special Issue: Machine Learning-Guided Intelligent Modeling with Its Industrial Applications)
Computer Modeling in Engineering & Sciences 2024, 138(1), 459-472. https://doi.org/10.32604/cmes.2023.029084
Received 31 January 2023; Accepted 24 April 2023; Issue published 22 September 2023
Abstract
Deep Convolutional Neural Networks (CNNs) have achieved high accuracy in image classification tasks, however, most existing models are trained on high-quality images that are not subject to image degradation. In practice, images are often affected by various types of degradation which can significantly impact the performance of CNNs. In this work, we investigate the influence of image degradation on three typical image classification CNNs and propose a Degradation Type Adaptive Image Classification Model (DTA-ICM) to improve the existing CNNs’ classification accuracy on degraded images. The proposed DTA-ICM comprises two key components: a Degradation Type Predictor (DTP) and a Degradation Type Specified Image Classifier (DTS-IC) set, which is trained on existing CNNs for specified types of degradation. The DTP predicts the degradation type of a test image, and the corresponding DTS-IC is then selected to classify the image. We evaluate the performance of both the proposed DTP and the DTA-ICM on the Caltech 101 database. The experimental results demonstrate that the proposed DTP achieves an average accuracy of 99.70%. Moreover, the proposed DTA-ICM, based on AlexNet, VGG19, and ResNet152, exhibits an average accuracy improvement of 20.63%, 18.22%, and 12.9%, respectively, compared with the original CNNs in classifying degraded images. It suggests that the proposed DTA-ICM can effectively improve the classification performance of existing CNNs on degraded images, which has important practical implications.Keywords
Cite This Article
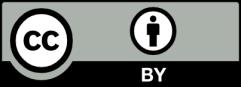