Open Access
ARTICLE
Bearing Fault Diagnosis Based on Deep Discriminative Adversarial Domain Adaptation Neural Networks
1 School of Mechanical and Equipment Engineering, Hebei University of Engineering, Handan, 056000, China
2 College of Mechanical Engineering, Anyang Institute of Technology, Anyang, 455000, China
3 Department of Mechanical and Electrical Engineering, Yuncheng University, Yuncheng, 044000, China
* Corresponding Author: Jie Wu. Email:
(This article belongs to the Special Issue: Computer-Aided Uncertainty Modeling and Reliability Evaluation for Complex Engineering Structures)
Computer Modeling in Engineering & Sciences 2024, 138(3), 2619-2640. https://doi.org/10.32604/cmes.2023.031360
Received 06 June 2023; Accepted 18 July 2023; Issue published 15 December 2023
Abstract
Intelligent diagnosis driven by big data for mechanical fault is an important means to ensure the safe operation of equipment. In these methods, deep learning-based machinery fault diagnosis approaches have received increasing attention and achieved some results. It might lead to insufficient performance for using transfer learning alone and cause misclassification of target samples for domain bias when building deep models to learn domain-invariant features. To address the above problems, a deep discriminative adversarial domain adaptation neural network for the bearing fault diagnosis model is proposed (DDADAN). In this method, the raw vibration data are firstly converted into frequency domain data by Fast Fourier Transform, and an improved deep convolutional neural network with wide first-layer kernels is used as a feature extractor to extract deep fault features. Then, domain invariant features are learned from the fault data with correlation alignment-based domain adversarial training. Furthermore, to enhance the discriminative property of features, discriminative feature learning is embedded into this network to make the features compact, as well as separable between classes within the class. Finally, the performance and anti-noise capability of the proposed method are evaluated using two sets of bearing fault datasets. The results demonstrate that the proposed method is capable of handling domain offset caused by different working conditions and maintaining more than 97.53% accuracy on various transfer tasks. Furthermore, the proposed method can achieve high diagnostic accuracy under varying noise levels.Keywords
Cite This Article
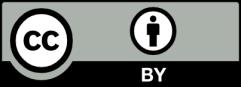
This work is licensed under a Creative Commons Attribution 4.0 International License , which permits unrestricted use, distribution, and reproduction in any medium, provided the original work is properly cited.