Open Access
ARTICLE
Distributed Stochastic Optimization with Compression for Non-Strongly Convex Objectives
School of Information Science and Technology, Fudan University, Shanghai, China
* Corresponding Author: Yuedong Xu. Email:
Computer Modeling in Engineering & Sciences 2024, 139(1), 459-481. https://doi.org/10.32604/cmes.2023.043247
Received 26 June 2023; Accepted 20 October 2023; Issue published 30 December 2023
Abstract
We are investigating the distributed optimization problem, where a network of nodes works together to minimize a global objective that is a finite sum of their stored local functions. Since nodes exchange optimization parameters through the wireless network, large-scale training models can create communication bottlenecks, resulting in slower training times. To address this issue, CHOCO-SGD was proposed, which allows compressing information with arbitrary precision without reducing the convergence rate for strongly convex objective functions. Nevertheless, most convex functions are not strongly convex (such as logistic regression or Lasso), which raises the question of whether this algorithm can be applied to non-strongly convex functions. In this paper, we provide the first theoretical analysis of the convergence rate of CHOCO-SGD on non-strongly convex objectives. We derive a sufficient condition, which limits the fidelity of compression, to guarantee convergence. Moreover, our analysis demonstrates that within the fidelity threshold, this algorithm can significantly reduce transmission burden while maintaining the same convergence rate order as its no-compression equivalent. Numerical experiments further validate the theoretical findings by demonstrating that CHOCO-SGD improves communication efficiency and keeps the same convergence rate order simultaneously. And experiments also show that the algorithm fails to converge with low compression fidelity and in time-varying topologies. Overall, our study offers valuable insights into the potential applicability of CHOCO-SGD for non-strongly convex objectives. Additionally, we provide practical guidelines for researchers seeking to utilize this algorithm in real-world scenarios.Keywords
Cite This Article
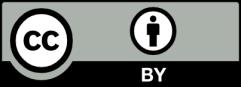