Open Access
ARTICLE
Cross-Dimension Attentive Feature Fusion Network for Unsupervised Time-Series Anomaly Detection
1 School of Computer Science and Engineering, University of Electronic Science and Technology of China, Chengdu, 611731, China
2 School of Computer and Software Engineering, Xihua University, Chengdu, 610039, China
3 School of Computer Science, Sichuan University, Chengdu, 610065, China
4 National Innovation Center for UHD Video Technology, Chengdu, 610095, China
* Corresponding Author: Yao Zhou. Email:
(This article belongs to the Special Issue: Machine Learning Empowered Distributed Computing: Advance in Architecture, Theory and Practice)
Computer Modeling in Engineering & Sciences 2024, 139(3), 3011-3027. https://doi.org/10.32604/cmes.2023.047065
Received 23 October 2023; Accepted 21 December 2023; Issue published 11 March 2024
Abstract
Time series anomaly detection is crucial in various industrial applications to identify unusual behaviors within the time series data. Due to the challenges associated with annotating anomaly events, time series reconstruction has become a prevalent approach for unsupervised anomaly detection. However, effectively learning representations and achieving accurate detection results remain challenging due to the intricate temporal patterns and dependencies in real-world time series. In this paper, we propose a cross-dimension attentive feature fusion network for time series anomaly detection, referred to as CAFFN. Specifically, a series and feature mixing block is introduced to learn representations in 1D space. Additionally, a fast Fourier transform is employed to convert the time series into 2D space, providing the capability for 2D feature extraction. Finally, a cross-dimension attentive feature fusion mechanism is designed that adaptively integrates features across different dimensions for anomaly detection. Experimental results on real-world time series datasets demonstrate that CAFFN performs better than other competing methods in time series anomaly detection.Keywords
Cite This Article
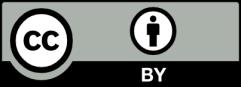