Open Access
ARTICLE
A Lightweight Network with Dual Encoder and Cross Feature Fusion for Cement Pavement Crack Detection
1 School of Computer Science and Technology, Chongqing University of Posts and Telecommunications, Chongqing, 400065, China
2 School of Software Engineering, Chongqing University of Posts and Telecommunications, Chongqing, 400065, China
* Corresponding Author: Zhong Qu. Email:
Computer Modeling in Engineering & Sciences 2024, 140(1), 255-273. https://doi.org/10.32604/cmes.2024.048175
Received 29 November 2023; Accepted 23 January 2024; Issue published 16 April 2024
Abstract
Automatic crack detection of cement pavement chiefly benefits from the rapid development of deep learning, with convolutional neural networks (CNN) playing an important role in this field. However, as the performance of crack detection in cement pavement improves, the depth and width of the network structure are significantly increased, which necessitates more computing power and storage space. This limitation hampers the practical implementation of crack detection models on various platforms, particularly portable devices like small mobile devices. To solve these problems, we propose a dual-encoder-based network architecture that focuses on extracting more comprehensive fracture feature information and combines cross-fusion modules and coordinated attention mechanisms for more efficient feature fusion. Firstly, we use small channel convolution to construct shallow feature extraction module (SFEM) to extract low-level feature information of cracks in cement pavement images, in order to obtain more information about cracks in the shallow features of images. In addition, we construct large kernel atrous convolution (LKAC) to enhance crack information, which incorporates coordination attention mechanism for non-crack information filtering, and large kernel atrous convolution with different cores, using different receptive fields to extract more detailed edge and context information. Finally, the three-stage feature map outputs from the shallow feature extraction module is cross-fused with the two-stage feature map outputs from the large kernel atrous convolution module, and the shallow feature and detailed edge feature are fully fused to obtain the final crack prediction map. We evaluate our method on three public crack datasets: DeepCrack, CFD, and Crack500. Experimental results on the DeepCrack dataset demonstrate the effectiveness of our proposed method compared to state-of-the-art crack detection methods, which achieves Precision (P) 87.2%, Recall (R) 87.7%, and F-score () 87.4%. Thanks to our lightweight crack detection model, the parameter count of the model in real-world detection scenarios has been significantly reduced to less than 2M. This advancement also facilitates technical support for portable scene detection.Graphic Abstract
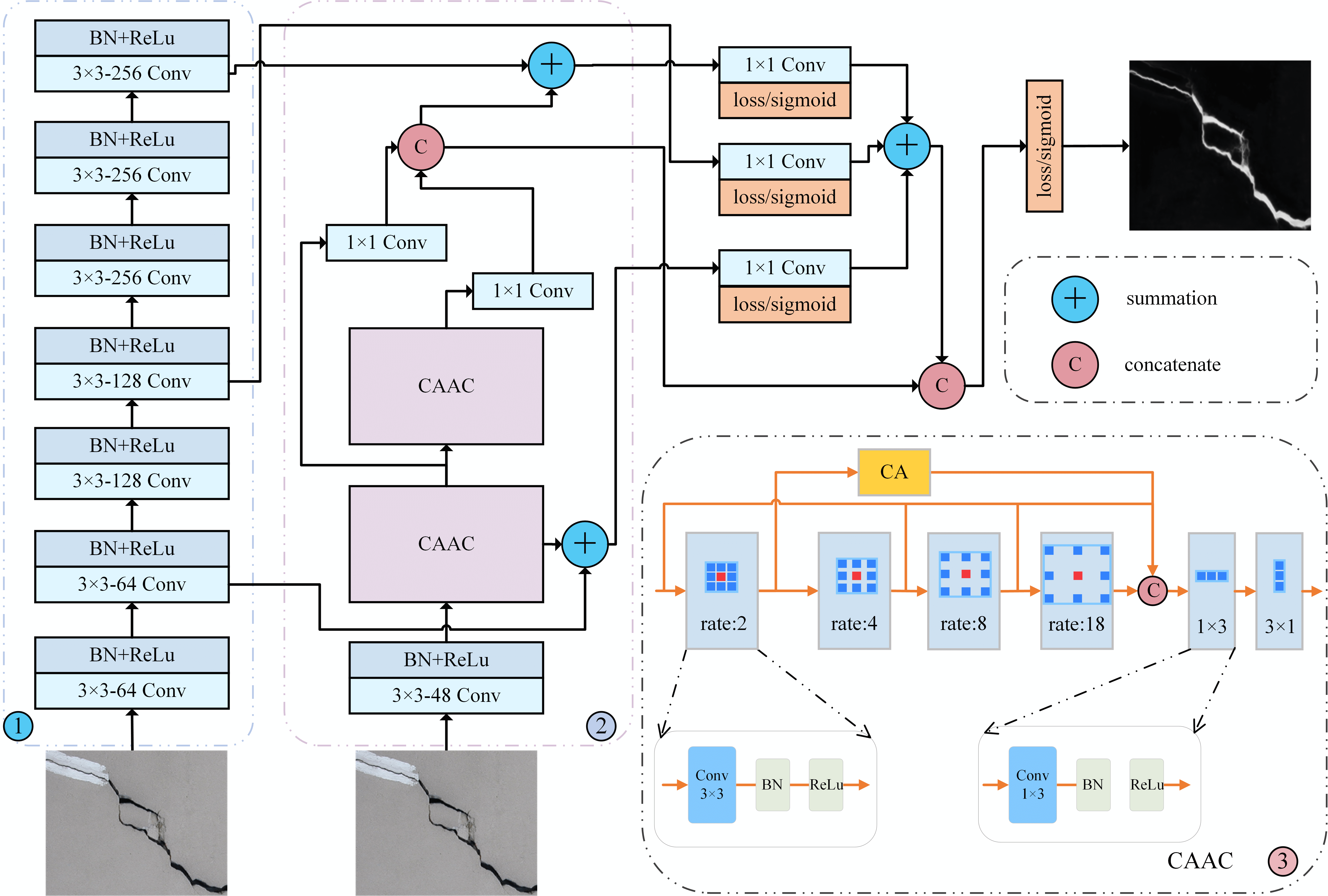
Keywords
Cite This Article
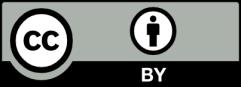