Open Access
ARTICLE
Disease Recognition of Apple Leaf Using Lightweight Multi-Scale Network with ECANet
College of Information Technology, Jilin Agricultural University, Changchun, 130118, China
* Corresponding Author: Chunguang Bi. Email:
(This article belongs to the Special Issue: Swarm Intelligence and Applications in Combinatorial Optimization)
Computer Modeling in Engineering & Sciences 2022, 132(3), 711-738. https://doi.org/10.32604/cmes.2022.020263
Received 13 November 2021; Accepted 27 January 2022; Issue published 27 June 2022
Abstract
To solve the problem of difficulty in identifying apple diseases in the natural environment and the low application rate of deep learning recognition networks, a lightweight ResNet (LW-ResNet) model for apple disease recognition is proposed. Based on the deep residual network (ResNet18), the multi-scale feature extraction layer is constructed by group convolution to realize the compression model and improve the extraction ability of different sizes of lesion features. By improving the identity mapping structure to reduce information loss. By introducing the efficient channel attention module (ECANet) to suppress noise from a complex background. The experimental results show that the average precision, recall and F1-score of the LW-ResNet on the test set are 97.80%, 97.92% and 97.85%, respectively. The parameter memory is 2.32 MB, which is 94% less than that of ResNet18. Compared with the classic lightweight networks SqueezeNet and MobileNetV2, LW-ResNet has obvious advantages in recognition performance, speed, parameter memory requirement and time complexity. The proposed model has the advantages of low computational cost, low storage cost, strong real-time performance, high identification accuracy, and strong practicability, which can meet the needs of real-time identification task of apple leaf disease on resource-constrained devices.Keywords
Cite This Article
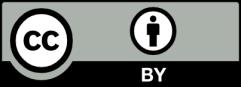