Open Access
ARTICLE
Large-Scale Multi-Objective Optimization Algorithm Based on Weighted Overlapping Grouping of Decision Variables
1 Shanxi Key Laboratory of Big Data Analysis and Parallel Computing, Taiyuan University of Science and Technology, Taiyuan, 030024, China
2 School of State Key Laboratory of Novel Software Technology, Nanjing University, Nanjing, 210008, China
* Corresponding Author: Xingjuan Cai. Email:
Computer Modeling in Engineering & Sciences 2024, 140(1), 363-383. https://doi.org/10.32604/cmes.2024.049044
Received 26 December 2023; Accepted 22 February 2024; Issue published 16 April 2024
Abstract
The large-scale multi-objective optimization algorithm (LSMOA), based on the grouping of decision variables, is an advanced method for handling high-dimensional decision variables. However, in practical problems, the interaction among decision variables is intricate, leading to large group sizes and suboptimal optimization effects; hence a large-scale multi-objective optimization algorithm based on weighted overlapping grouping of decision variables (MOEAWOD) is proposed in this paper. Initially, the decision variables are perturbed and categorized into convergence and diversity variables; subsequently, the convergence variables are subdivided into groups based on the interactions among different decision variables. If the size of a group surpasses the set threshold, that group undergoes a process of weighting and overlapping grouping. Specifically, the interaction strength is evaluated based on the interaction frequency and number of objectives among various decision variables. The decision variable with the highest interaction in the group is identified and disregarded, and the remaining variables are then reclassified into subgroups. Finally, the decision variable with the strongest interaction is added to each subgroup. MOEAWOD minimizes the interactivity between different groups and maximizes the interactivity of decision variables within groups, which contributed to the optimized direction of convergence and diversity exploration with different groups. MOEAWOD was subjected to testing on 18 benchmark large-scale optimization problems, and the experimental results demonstrate the effectiveness of our methods. Compared with the other algorithms, our method is still at an advantage.Keywords
Cite This Article
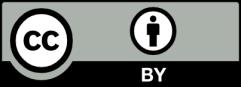