Open Access
ARTICLE
Determination of the Pile Drivability Using Random Forest Optimized by Particle Swarm Optimization and Bayesian Optimizer
1 State Key Laboratory of Eco-Hydraulics in Northwest Arid Region, Xi’an University of Technology, Xi’an, 710048, China
2 School of Resources and Safety Engineering, Central South University, Changsha, 410083, China
* Corresponding Authors: Juncheng Gao. Email: ; Hongning Qi. Email:
(This article belongs to the Special Issue: Computational Intelligent Systems for Solving Complex Engineering Problems: Principles and Applications-II)
Computer Modeling in Engineering & Sciences 2024, 141(1), 871-892. https://doi.org/10.32604/cmes.2024.052830
Received 16 April 2024; Accepted 11 June 2024; Issue published 20 August 2024
Abstract
Driven piles are used in many geological environments as a practical and convenient structural component. Hence, the determination of the drivability of piles is actually of great importance in complex geotechnical applications. Conventional methods of predicting pile drivability often rely on simplified physical models or empirical formulas, which may lack accuracy or applicability in complex geological conditions. Therefore, this study presents a practical machine learning approach, namely a Random Forest (RF) optimized by Bayesian Optimization (BO) and Particle Swarm Optimization (PSO), which not only enhances prediction accuracy but also better adapts to varying geological environments to predict the drivability parameters of piles (i.e., maximum compressive stress, maximum tensile stress, and blow per foot). In addition, support vector regression, extreme gradient boosting, k nearest neighbor, and decision tree are also used and applied for comparison purposes. In order to train and test these models, among the 4072 datasets collected with 17 model inputs, 3258 datasets were randomly selected for training, and the remaining 814 datasets were used for model testing. Lastly, the results of these models were compared and evaluated using two performance indices, i.e., the root mean square error (RMSE) and the coefficient of determination (R). The results indicate that the optimized RF model achieved lower RMSE than other prediction models in predicting the three parameters, specifically 0.044, 0.438, and 0.146; and higher R² values than other implemented techniques, specifically 0.966, 0.884, and 0.977. In addition, the sensitivity and uncertainty of the optimized RF model were analyzed using Sobol sensitivity analysis and Monte Carlo (MC) simulation. It can be concluded that the optimized RF model could be used to predict the performance of the pile, and it may provide a useful reference for solving some problems under similar engineering conditions.Graphic Abstract
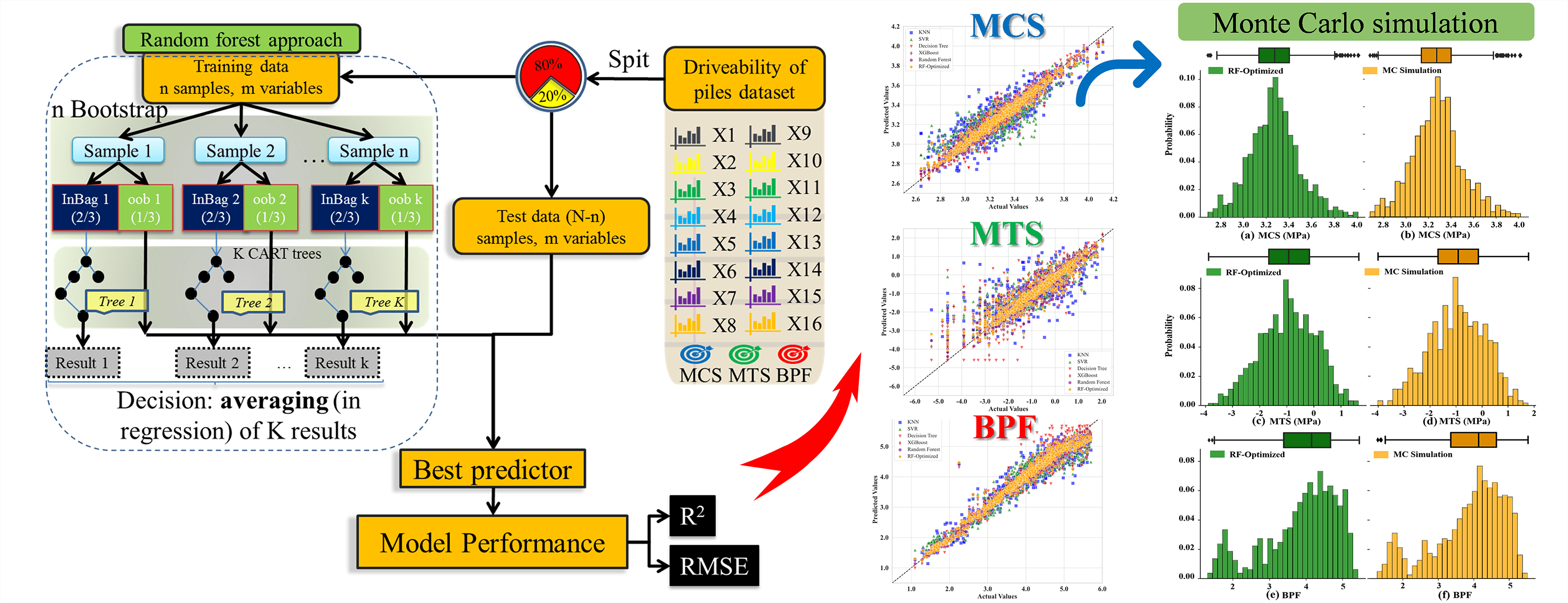
Keywords
Cite This Article
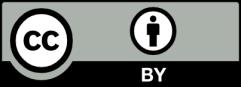
This work is licensed under a Creative Commons Attribution 4.0 International License , which permits unrestricted use, distribution, and reproduction in any medium, provided the original work is properly cited.