Open Access
ARTICLE
An Incremental Kriging Method for Sequential Optimal Experimental Design
National CAD Supported Software Engineering Centre in Huazhong University of Science and Technology, Wuhan, 430074, Hubei, P.R. China
School of Electrical Engineering & Mechano-Electronic Engineering in Xuchang University, Xuchang, 461000, Henan, P.R. China.
Corresponding author. Email: cad.wyz@mail.hust.edu.cn
Computer Modeling in Engineering & Sciences 2014, 97(4), 323-357. https://doi.org/10.3970/cmes.2014.097.323
Abstract
Kriging model, which provides an exact interpolation and minimizes the error estimates, is a highly-precise global approximation model in contrast with other traditional response surfaces. Therefore, sequential exploratory experimental design (SEED) with Kriging model is crucial for globally approximating a complex black-box function. However, the more sampling points are, the longer time it would take to update the Kriging model during sequential exploratory design. This paper, therefore, proposes a new construction method called incremental Kriging method (IKM) to improve the constructing efficiency with just a little and controllable loss of accuracy for Kriging model. The IKM, based on the matrix segmentation theory, is under the premise that the correlated parameter θ remains unchanged. Meantime, it utilizes the original model and incremental sampling data to quickly obtain an updated Kriging model. Fortunately, a large number of numerical tests showed that the stability of parameter θ would become better and better with the continual increase of the new sampling points in most instances. Even if there is a slight change for value θ, there is not obvious effect on the accuracy of Kriging model. Then, a new sequential incremental experimental design (SIED) algorithm based on IKM is presented to construct Kriging model steadily and effectively. At each sampling step, the SIED method finds an optimal sampling point which maximizes the mean square error of the current model. Meantime, it judges whether the θ should be changed according to the updating criterion. Kriging model will be updated by IKM when θ remains unchanged, or recreates the model with all the sampling points, otherwise. Finally, seven numerical tests and three engineering examples are given to illustrate the applicability, effectiveness and superiority of the proposed methods.Keywords
Cite This Article
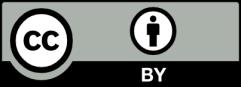