Open Access
ARTICLE
A New Adaptive Regularization Parameter Selection Based on Expected Patch Log Likelihood
1 School of Math and Statistics, Nanjing University of Information Science and Technology, Nanjing, 210044, China.
* Corresponding Author: Shunfeng Wang. Email: .
Journal of Cyber Security 2020, 2(1), 25-36. https://doi.org/10.32604/jcs.2020.06429
Abstract
Digital images have been applied to various areas such as evidence in courts. However, it always suffers from noise by criminals. This type of computer network security has become a hot issue that can’t be ignored. In this paper, we focus on noise removal so as to provide guarantees for computer network security. Firstly, we introduce a well-known denoising method called Expected Patch Log Likelihood (EPLL) with Gaussian Mixture Model as its prior. This method achieves exciting results in noise removal. However, there remain problems to be solved such as preserving the edge and meaningful details in image denoising, cause it considers a constant as regularization parameter so that we denoise with the same strength on the whole image. This leads to a problem that edges and meaningful details may be oversmoothed. Under the consideration of preserving edges of the image, we introduce a new adaptive parameter selection based on EPLL by the use of the image gradient and variance, which varies with different regions of the image. Moreover, we add a gradient fidelity term to relieve staircase effect and preserve more details. The experiment shows that our proposed method proves the effectiveness not only in vision but also on quantitative evaluation.Keywords
Cite This Article
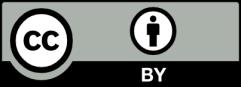