Open Access
ARTICLE
Clustering building morphometrics using national spatial data
1. ESPACE, Université Côte d’Azur, CNRS
98, bd Herriot, BP 3209, 06200 Nice, France
{alessandro.araldi, giovanni.fusco@univ-cotedazur.fr
2. KCITYLABS - Kinaxia Group
80, route des Lucioles, 06560 Valbonne, France
{david.emsellem, denis.overal]@kcitylabs.fr
3. I3S, Inria, CNRS, Université Côte d’Azur
2000, route des Lucioles, 06900 Sophia Antipolis, France
andrea.tettamanzi@univ-cotedazur.fr
Revue Internationale de Géomatique 2022, 31(2), 265-302. https://doi.org/10.3166/RIG.31.265-302© 2022
Abstract
The identification and description of building typologies play a fundamental role in the understanding of the overall built-up form. A growing body of research is developing and implementing sophisticated, computer-aided protocols for the identification of building typologies. This paper shares the same goal. An innovative data-driven procedure for the unsupervised identification and description of building types and organization is here presented. After a specific pre-processing procedure, we develop an unsupervised clustering combining a new algorithm of Naive Bayes inference and hierarchical ascendant approaches relying on six morphometric features of buildings. This protocol allows us to identify groups of buildings sharing specific similar morphological characteristics and their overall structure at different aggregation levels. The proposed methodology is implemented and evaluated on the overall ordinary (e.g. notspecialized) building stock of France.Keywords
Cite This Article
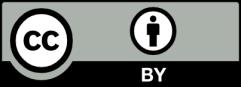