Open Access
ARTICLE
Multimodal Social Media Fake News Detection Based on Similarity Inference and Adversarial Networks
1 College of Computer, Zhongyuan University of Technology, Zhengzhou, 450007, China
2 Henan Key Laboratory of Cyberspace Situation Awareness, Zhengzhou 450001, China
* Corresponding Author: Fangfang Shan. Email:
Computers, Materials & Continua 2024, 79(1), 581-605. https://doi.org/10.32604/cmc.2024.046202
Received 22 September 2023; Accepted 23 February 2024; Issue published 25 April 2024
Abstract
As social networks become increasingly complex, contemporary fake news often includes textual descriptions of events accompanied by corresponding images or videos. Fake news in multiple modalities is more likely to create a misleading perception among users. While early research primarily focused on text-based features for fake news detection mechanisms, there has been relatively limited exploration of learning shared representations in multimodal (text and visual) contexts. To address these limitations, this paper introduces a multimodal model for detecting fake news, which relies on similarity reasoning and adversarial networks. The model employs Bidirectional Encoder Representation from Transformers (BERT) and Text Convolutional Neural Network (Text-CNN) for extracting textual features while utilizing the pre-trained Visual Geometry Group 19-layer (VGG-19) to extract visual features. Subsequently, the model establishes similarity representations between the textual features extracted by Text-CNN and visual features through similarity learning and reasoning. Finally, these features are fused to enhance the accuracy of fake news detection, and adversarial networks have been employed to investigate the relationship between fake news and events. This paper validates the proposed model using publicly available multimodal datasets from Weibo and Twitter. Experimental results demonstrate that our proposed approach achieves superior performance on Twitter, with an accuracy of 86%, surpassing traditional unimodal modal models and existing multimodal models. In contrast, the overall better performance of our model on the Weibo dataset surpasses the benchmark models across multiple metrics. The application of similarity reasoning and adversarial networks in multimodal fake news detection significantly enhances detection effectiveness in this paper. However, current research is limited to the fusion of only text and image modalities. Future research directions should aim to further integrate features from additional modalities to comprehensively represent the multifaceted information of fake news.Keywords
Cite This Article
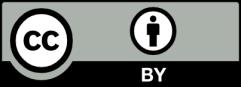