Open Access
ARTICLE
Generating Questions Based on Semi-Automated and End-to-End Neural Network
The School of Information Engineering, Minzu University of China, Beijing, 100081, China.
Minority Languages Branch, National Language Resource and Monitoring Research Center, Beijing, 100081, China.
Queen’s University Belfast, Belfast, Northern Ireland, BT71NN, UK.
*Corresponding Author: Yuan Sun. Email: .
Computers, Materials & Continua 2019, 61(2), 617-628. https://doi.org/10.32604/cmc.2019.05860
Abstract
With the emergence of large-scale knowledge base, how to use triple information to generate natural questions is a key technology in question answering systems. The traditional way of generating questions require a lot of manual intervention and produce lots of noise. To solve these problems, we propose a joint model based on semi-automated model and End-to-End neural network to automatically generate questions. The semi-automated model can generate question templates and real questions combining the knowledge base and center graph. The End-to-End neural network directly sends the knowledge base and real questions to BiLSTM network. Meanwhile, the attention mechanism is utilized in the decoding layer, which makes the triples and generated questions more relevant. Finally, the experimental results on SimpleQuestions demonstrate the effectiveness of the proposed approach.Keywords
Cite This Article
Citations
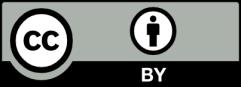