Open Access
ARTICLE
Combined CNN-LSTM Deep Learning Algorithms for Recognizing Human Physical Activities in Large and Distributed Manners: A Recommendation System
1 Control and Energy Management Laboratory, National Engineering School, Preparatory Institute for Engineering Studies, University of Sfax, Sfax, 3029, Tunisia
2 Higher Institute of Computer Science and Communication Technologies, University of Sousse, Sousse, BN1 9RH, Tunisia
3 College of Computer and Information Sciences, Jouf University, PO Box 2014, Sakakah, Saudi Arabia
* Corresponding Author: Alaa Alaerjan. Email:
(This article belongs to the Special Issue: The Next-generation Deep Learning Approaches to Emerging Real-world Applications)
Computers, Materials & Continua 2024, 79(1), 351-372. https://doi.org/10.32604/cmc.2024.048061
Received 26 November 2023; Accepted 05 February 2024; Issue published 25 April 2024
Abstract
Recognizing human activity (HAR) from data in a smartphone sensor plays an important role in the field of health to prevent chronic diseases. Daily and weekly physical activities are recorded on the smartphone and tell the user whether he is moving well or not. Typically, smartphones and their associated sensing devices operate in distributed and unstable environments. Therefore, collecting their data and extracting useful information is a significant challenge. In this context, the aim of this paper is twofold: The first is to analyze human behavior based on the recognition of physical activities. Using the results of physical activity detection and classification, the second part aims to develop a health recommendation system to notify smartphone users about their healthy physical behavior related to their physical activities. This system is based on the calculation of calories burned by each user during physical activities. In this way, conclusions can be drawn about a person’s physical behavior by estimating the number of calories burned after evaluating data collected daily or even weekly following a series of physical workouts. To identify and classify human behavior our methodology is based on artificial intelligence models specifically deep learning techniques like Long Short-Term Memory (LSTM), stacked LSTM, and bidirectional LSTM. Since human activity data contains both spatial and temporal information, we proposed, in this paper, to use of an architecture allowing the extraction of the two types of information simultaneously. While Convolutional Neural Networks (CNN) has an architecture designed for spatial information, our idea is to combine CNN with LSTM to increase classification accuracy by taking into consideration the extraction of both spatial and temporal data. The results obtained achieved an accuracy of 96%. On the other side, the data learned by these algorithms is prone to error and uncertainty. To overcome this constraint and improve performance (96%), we proposed to use the fusion mechanisms. The last combines deep learning classifiers to model non-accurate and ambiguous data to obtain synthetic information to aid in decision-making. The Voting and Dempster-Shafer (DS) approaches are employed. The results showed that fused classifiers based on DS theory outperformed individual classifiers (96%) with the highest accuracy level of 98%. Also, the findings disclosed that participants engaging in physical activities are healthy, showcasing a disparity in the distribution of physical activities between men and women.Keywords
Cite This Article
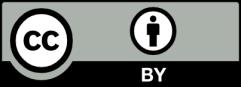