Open Access
ARTICLE
MIDNet: Deblurring Network for Material Microstructure Images
1 National Center for Materials Service Safety, University of Science and Technology Beijing, Beijing, 100083, China
2 School of Materials, Sun Yat-Sen University, Shenzhen, 518107, China
3 School of Materials Science and Engineering, Southern Marine Science and Engineering Guangdong Laboratory (Zhuhai), Sun Yat-Sen University, Guangzhou, 510006, China
* Corresponding Author: Dongbai Sun. Email:
(This article belongs to the Special Issue: Advances and Applications in Signal, Image and Video Processing)
Computers, Materials & Continua 2024, 79(1), 1187-1204. https://doi.org/10.32604/cmc.2024.046929
Received 19 October 2023; Accepted 20 December 2023; Issue published 25 April 2024
Abstract
Scanning electron microscopy (SEM) is a crucial tool in the field of materials science, providing valuable insights into the microstructural characteristics of materials. Unfortunately, SEM images often suffer from blurriness caused by improper hardware calibration or imaging automation errors, which present challenges in analyzing and interpreting material characteristics. Consequently, rectifying the blurring of these images assumes paramount significance to enable subsequent analysis. To address this issue, we introduce a Material Images Deblurring Network (MIDNet) built upon the foundation of the Nonlinear Activation Free Network (NAFNet). MIDNet is meticulously tailored to address the blurring in images capturing the microstructure of materials. The key contributions include enhancing the NAFNet architecture for better feature extraction and representation, integrating a novel soft attention mechanism to uncover important correlations between encoder and decoder, and introducing new multi-loss functions to improve training effectiveness and overall model performance. We conduct a comprehensive set of experiments utilizing the material blurry dataset and compare them to several state-of-the-art deblurring methods. The experimental results demonstrate the applicability and effectiveness of MIDNet in the domain of deblurring material microstructure images, with a PSNR (Peak Signal-to-Noise Ratio) reaching 35.26 dB and an SSIM (Structural Similarity) of 0.946. Our dataset is available at: .Keywords
Cite This Article
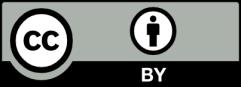