Open Access
ARTICLE
Leveraging User-Generated Comments and Fused BiLSTM Models to Detect and Predict Issues with Mobile Apps
Department of Computer Science, College of Computer Science and Engineering, Taibah University, Medina, 42353, Saudi Arabia
* Corresponding Author: Wael M. S. Yafooz. Email:
(This article belongs to the Special Issue: Optimization for Artificial Intelligence Application)
Computers, Materials & Continua 2024, 79(1), 735-759. https://doi.org/10.32604/cmc.2024.048270
Received 02 December 2023; Accepted 26 February 2024; Issue published 25 April 2024
Abstract
In the last decade, technical advancements and faster Internet speeds have also led to an increasing number of mobile devices and users. Thus, all contributors to society, whether young or old members, can use these mobile apps. The use of these apps eases our daily lives, and all customers who need any type of service can access it easily, comfortably, and efficiently through mobile apps. Particularly, Saudi Arabia greatly depends on digital services to assist people and visitors. Such mobile devices are used in organizing daily work schedules and services, particularly during two large occasions, Umrah and Hajj. However, pilgrims encounter mobile app issues such as slowness, conflict, unreliability, or user-unfriendliness. Pilgrims comment on these issues on mobile app platforms through reviews of their experiences with these digital services. Scholars have made several attempts to solve such mobile issues by reporting bugs or non-functional requirements by utilizing user comments. However, solving such issues is a great challenge, and the issues still exist. Therefore, this study aims to propose a hybrid deep learning model to classify and predict mobile app software issues encountered by millions of pilgrims during the Hajj and Umrah periods from the user perspective. Firstly, a dataset was constructed using user-generated comments from relevant mobile apps using natural language processing methods, including information extraction, the annotation process, and pre-processing steps, considering a multi-class classification problem. Then, several experiments were conducted using common machine learning classifiers, Artificial Neural Networks (ANN), Long Short-Term Memory (LSTM), and Convolutional Neural Network Long Short-Term Memory (CNN-LSTM) architectures, to examine the performance of the proposed model. Results show 96% in F1-score and accuracy, and the proposed model outperformed the mentioned models.Keywords
Cite This Article
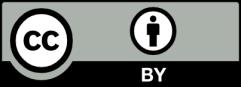