Open Access
ARTICLE
MAIPFE: An Efficient Multimodal Approach Integrating Pre-Emptive Analysis, Personalized Feature Selection, and Explainable AI
1 School of Computer Science and Engineering, VIT-AP University, Amaravathi, Andhra Pradesh, 522241, India
* Corresponding Author: S. Gopikrishnan. Email:
Computers, Materials & Continua 2024, 79(2), 2229-2251. https://doi.org/10.32604/cmc.2024.047438
Received 06 November 2023; Accepted 08 January 2024; Issue published 15 May 2024
Abstract
Medical Internet of Things (IoT) devices are becoming more and more common in healthcare. This has created a huge need for advanced predictive health modeling strategies that can make good use of the growing amount of multimodal data to find potential health risks early and help individuals in a personalized way. Existing methods, while useful, have limitations in predictive accuracy, delay, personalization, and user interpretability, requiring a more comprehensive and efficient approach to harness modern medical IoT devices. MAIPFE is a multimodal approach integrating pre-emptive analysis, personalized feature selection, and explainable AI for real-time health monitoring and disease detection. By using AI for early disease detection, personalized health recommendations, and transparency, healthcare will be transformed. The Multimodal Approach Integrating Pre-emptive Analysis, Personalized Feature Selection, and Explainable AI (MAIPFE) framework, which combines Firefly Optimizer, Recurrent Neural Network (RNN), Fuzzy C Means (FCM), and Explainable AI, improves disease detection precision over existing methods. Comprehensive metrics show the model’s superiority in real-time health analysis. The proposed framework outperformed existing models by 8.3% in disease detection classification precision, 8.5% in accuracy, 5.5% in recall, 2.9% in specificity, 4.5% in AUC (Area Under the Curve), and 4.9% in delay reduction. Disease prediction precision increased by 4.5%, accuracy by 3.9%, recall by 2.5%, specificity by 3.5%, AUC by 1.9%, and delay levels decreased by 9.4%. MAIPFE can revolutionize healthcare with preemptive analysis, personalized health insights, and actionable recommendations. The research shows that this innovative approach improves patient outcomes and healthcare efficiency in the real world.Keywords
Cite This Article
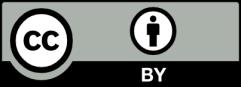