Open Access
ARTICLE
Paragraph Vector Representation Based on Word to Vector and CNN Learning
College of Computer, National University of Defense Technology, De Ya Road, Changsha 410073, China.
School of Computer Science, Simon Fraser University, 8888 UNIVERSITY DRIVE, BURNABY, BC, V5A 1S6, Vancouver, Canada.
* Corresponding author: Zeyu Xiong. Email: .
Computers, Materials & Continua 2018, 55(2), 213-227. https://doi.org/10.3970/cmc.2018.01762
Abstract
Document processing in natural language includes retrieval, sentiment analysis, theme extraction, etc. Classical methods for handling these tasks are based on models of probability, semantics and networks for machine learning. The probability model is loss of semantic information in essential, and it influences the processing accuracy. Machine learning approaches include supervised, unsupervised, and semi-supervised approaches, labeled corpora is necessary for semantics model and supervised learning. The method for achieving a reliably labeled corpus is done manually, it is costly and time-consuming because people have to read each document and annotate the label of each document. Recently, the continuous CBOW model is efficient for learning high-quality distributed vector representations, and it can capture a large number of precise syntactic and semantic word relationships, this model can be easily extended to learn paragraph vector, but it is not precise. Towards these problems, this paper is devoted to developing a new model for learning paragraph vector, we combine the CBOW model and CNNs to establish a new deep learning model. Experimental results show that paragraph vector generated by the new model is better than the paragraph vector generated by CBOW model in semantic relativeness and accuracy.Keywords
Cite This Article
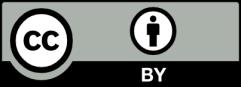