Open Access
ARTICLE
Feature Selection Method Based on Class Discriminative Degree for Intelligent Medical Diagnosis
College of Computer, National University of Defense Technology, Changsha 410073, China.
Computer Science and Technology, Central South University, Changsha 999078, China .
School of Data and Computer Science, Sun Yat-sen University, Guangzhou 510006, China.
Faculty of Information Technology, Macau University of Science and Technology, MACAU 410073, MO.
Xuzhou University of Technology, Xuzhou 221002, China .
* Corresponding Author: Zhiping Cai. Email: .
Computers, Materials & Continua 2018, 55(3), 419-433. https://doi.org/10.3970/cmc.2018.02289
Abstract
By using efficient and timely medical diagnostic decision making, clinicians can positively impact the quality and cost of medical care. However, the high similarity of clinical manifestations between diseases and the limitation of clinicians’ knowledge both bring much difficulty to decision making in diagnosis. Therefore, building a decision support system that can assist medical staff in diagnosing and treating diseases has lately received growing attentions in the medical domain. In this paper, we employ a multi-label classification framework to classify the Chinese electronic medical records to establish corresponding relation between the medical records and disease categories, and compare this method with the traditional medical expert system to verify the performance. To select the best subset of patient features, we propose a feature selection method based on the composition and distribution of symptoms in electronic medical records and compare it with the traditional feature selection methods such as chi-square test. We evaluate the feature selection methods and diagnostic models from two aspects, false negative rate (FNR) and accuracy. Extensive experiments have conducted on a real-world Chinese electronic medical record database. The evaluation results demonstrate that our proposed feature selection method can improve the accuracy and reduce the FNR compare to the traditional feature selection methods, and the multi-label classification framework have better accuracy and lower FNR than the traditional expert system.Keywords
Cite This Article
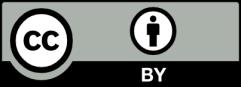