Open Access
ARTICLE
An Empirical Comparison on Multi-Target Regression Learning
School of Electronic and Information Engineering, Suzhou University of Science and Technology, Su zhou, China.
Department of Computer Science, University of Central Arkansas, Conway, AR 72035, USA.
* Corresponding Author: Victor S. Sheng. Email: .
Computers, Materials & Continua 2018, 56(2), 185-198. https://doi.org/10.3970/cmc.2018.03694
Abstract
Multi-target regression is concerned with the simultaneous prediction of multiple continuous target variables based on the same set of input variables. It has received relatively small attention from the Machine Learning community. However, multi-target regression exists in many real-world applications. In this paper we conduct extensive experiments to investigate the performance of three representative multi-target regression learning algorithms (i.e. Multi-Target Stacking (MTS), Random Linear Target Combination (RLTC), and Multi-Objective Random Forest (MORF)), comparing the baseline single-target learning. Our experimental results show that all three multi-target regression learning algorithms do improve the performance of the single-target learning. Among them, MTS performs the best, followed by RLTC, followed by MORF. However, the single-target learning sometimes still performs very well, even the best. This analysis sheds the light on multi-target regression learning and indicates that the single-target learning is a competitive baseline for multi-target regression learning on multi-target domains.Keywords
Cite This Article
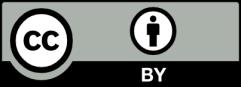