Open Access
ARTICLE
An Evidence Combination Method based on DBSCAN Clustering
College of Computer Science and Electronic Engineering, and Key Laboratory for Embedded and Network Computing of Hunan Province, Hunan University, Changsha, Hunan, 410082, China.
Department of Electrical and Computer Engineering, Virginia Polytechnic Institute and State University, Virginia, Blacksburg, 24060, USA.
* Corresponding Author: Kehua Yang. Email: .
Computers, Materials & Continua 2018, 57(2), 269-281. https://doi.org/10.32604/cmc.2018.03696
Abstract
Dempster-Shafer (D-S) evidence theory is a key technology for integrating uncertain information from multiple sources. However, the combination rules can be paradoxical when the evidence seriously conflict with each other. In the paper, we propose a novel combination algorithm based on unsupervised Density-Based Spatial Clustering of Applications with Noise (DBSCAN) density clustering. In the proposed mechanism, firstly, the original evidence sets are preprocessed by DBSCAN density clustering, and a successfully focal element similarity criteria is used to mine the potential information between the evidence, and make a correct measure of the conflict evidence. Then, two different discount factors are adopted to revise the original evidence sets, based on the result of DBSCAN density clustering. Finally, we conduct the information fusion for the revised evidence sets by D-S combination rules. Simulation results show that the proposed method can effectively solve the synthesis problem of high-conflict evidence, with better accuracy, stability and convergence speed.Keywords
Cite This Article
Citations
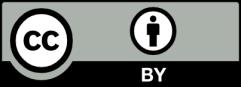