Open Access
ARTICLE
Symmetric Learning Data Augmentation Model for Underwater Target Noise Data Expansion
College of Computer Science and Technology, Harbin Engineering University, Harbin , 150001, China.
College of Computer and Information Engineering, Heilongjiang University of Science and Technology, Harbin, 150022, China.
School of Software and Microelectronics, Harbin University of Science and Technology, Harbin, 150080, China.
Department of Computer Science and Information Systems, University of Limerick, Limerick, Ireland .
* Corresponding Author: Hongbin Wang. Email: .
Computers, Materials & Continua 2018, 57(3), 521-532. https://doi.org/10.32604/cmc.2018.03710
Abstract
An important issue for deep learning models is the acquisition of training of data. Without abundant data from a real production environment for training, deep learning models would not be as widely used as they are today. However, the cost of obtaining abundant real-world environment is high, especially for underwater environments. It is more straightforward to simulate data that is closed to that from real environment. In this paper, a simple and easy symmetric learning data augmentation model (SLDAM) is proposed for underwater target radiate-noise data expansion and generation. The SLDAM, taking the optimal classifier of an initial dataset as the discriminator, makes use of the structure of the classifier to construct a symmetric generator based on antagonistic generation. It generates data similar to the initial dataset that can be used to supplement training data sets. This model has taken into consideration feature loss and sample loss function in model training, and is able to reduce the dependence of the generation and expansion on the feature set. We verified that the SLDAM is able to data expansion with low calculation complexity. Our results showed that the SLDAM is able to generate new data without compromising data recognition accuracy, for practical application in a production environment.Keywords
Cite This Article
Citations
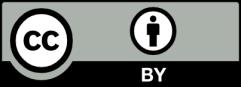
This work is licensed under a Creative Commons Attribution 4.0 International License , which permits unrestricted use, distribution, and reproduction in any medium, provided the original work is properly cited.