Open Access
ARTICLE
A Novel Ensemble Learning Algorithm Based on D-S Evidence Theory for IoT Security
College of Computer Science and Technology, Harbin Engineering University, Harbin , 150001, China
* Corresponding Author: Changting Shi. Email:
Computers, Materials & Continua 2018, 57(3), 635-652. https://doi.org/10.32604/cmc.2018.03754
Abstract
In the last decade, IoT has been widely used in smart cities, autonomous driving and Industry 4.0, which lead to improve efficiency, reliability, security and economic benefits. However, with the rapid development of new technologies, such as cognitive communication, cloud computing, quantum computing and big data, the IoT security is being confronted with a series of new threats and challenges. IoT device identification via Radio Frequency Fingerprinting (RFF) extracting from radio signals is a physical-layer method for IoT security. In physical-layer, RFF is a unique characteristic of IoT device themselves, which can difficultly be tampered. Just as people’s unique fingerprinting, different IoT devices exhibit different RFF which can be used for identification and authentication. In this paper, the structure of IoT device identification is proposed, the key technologies such as signal detection, RFF extraction, and classification model is discussed. Especially, based on the random forest and Dempster-Shafer evidence algorithm, a novel ensemble learning algorithm is proposed. Through theoretical modeling and experimental verification, the reliability and differentiability of RFF are extracted and verified, the classification result is shown under the real IoT device environments.Keywords
Cite This Article
Citations
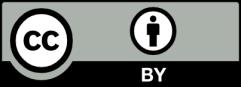