Open Access
ARTICLE
Yield Stress Prediction Model of RAFM Steel Based on the Improved GDM-SA-SVR Algorithm
School of Software, Central South University, Changsha, 410075, China.
School of Software, Central South University, Changsha, 410075, China.
Laboratory of Irradiation Effect, China Institute of Atomic Energy, Beijing, 102413, China.
* Corresponding Author: Ming Zhao. Email: .
Computers, Materials & Continua 2019, 58(3), 727-760. https://doi.org/10.32604/cmc.2019.04454
Abstract
With the development of society and the exhaustion of fossil energy, researcher need to identify new alternative energy sources. Nuclear energy is a very good choice, but the key to the successful application of nuclear technology is determined primarily by the behavior of nuclear materials in reactors. Therefore, we studied the radiation performance of the fusion material reduced activation ferritic/martensitic (RAFM) steel. The main novelty of this paper are the statistical analysis of RAFM steel data sets through related statistical analysis and the formula derivation of the gradient descent method (GDM) which combines the gradient descent search strategy of the Convex Optimization Theory to get the best value. Use GDM algorithm to upgrade the annealing stabilization process of simulated annealing algorithm. The yield stress performance of RAFM steel is successfully predicted by the hybrid model which is combined by simulated annealing (SA) with support vector machine (SVM) as the first time. The effect on yield stress by the main physical quantities such as irradiation temperature, irradiation dose and test temperature is also analyzed. The related prediction process is: first, we used the improved annealing algorithm to optimize the SVR model after training the SVR model on a training data set. Next, we established the yield stress prediction model of RAFM steel. The model can predict up to 96% of the data points with the prediction in the test set and the original data point in the 2σ range. The statistical test analysis shows that under the condition of confidence level α=0.01, the calculation results of the regression effect significance analysis pass the T-test.Keywords
Cite This Article
Citations
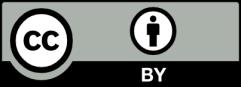