Open Access
ARTICLE
Computational Machine Learning Representation for the Flexoelectricity Effect in Truncated Pyramid Structures
Department of Computer Engineering, College of Computer and Information Sciences, King Saud University, Riyadh, Saudi Arabia.
Institute of Structural Mechanics, Bauhaus-Universität Weimar, Marienstr. 15, D-99423 Weimar, Germany.
Department of Mechanical Engineering, Arak University of Technology, 38181-41167 Arak, Iran.
Institute of Continuum Mechanics, Leibniz University Hannover, Hannover 30167, Germany.
Department of Geotechnical Engineering, Tongji University, Shanghai, 200092, China.
* Corresponding Author: Timon Rabczuk. Email: .
Computers, Materials & Continua 2019, 59(1), 79-87. https://doi.org/10.32604/cmc.2019.05882
Abstract
In this study, machine learning representation is introduced to evaluate the flexoelectricity effect in truncated pyramid nanostructure under compression. A Non-Uniform Rational B-spline (NURBS) based IGA formulation is employed to model the flexoelectricity. We investigate 2D system with an isotropic linear elastic material under plane strain conditions discretized by 45×30 grid of B-spline elements. Six input parameters are selected to construct a deep neural network (DNN) model. They are the Young's modulus, two dielectric permittivity constants, the longitudinal and transversal flexoelectric coefficients and the order of the shape function. The outputs of interest are the strain in the stress direction and the electric potential due flexoelectricity. The dataset are generated from the forward analysis of the flexoelectric model. 80% of the dataset is used for training purpose while the remaining is used for validation by checking the mean squared error. In addition to the input and output layers, the developed DNN model is composed of four hidden layers. The results showed high predictions capabilities of the proposed method with much lower computational time in comparison to the numerical model.Keywords
Cite This Article
Citations
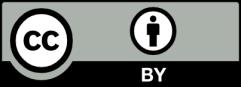