Open Access
ARTICLE
An Influence Maximization Algorithm Based on the Mixed Importance of Nodes
HuaiYin Institute of Technology, Huaian, 223003, China.
University of Fribourg, Fribourg, 1700, Switzerland.
* Corresponding Author: Bolun Chen. Email: .
Computers, Materials & Continua 2019, 59(2), 517-531. https://doi.org/10.32604/cmc.2019.05278
Abstract
The influence maximization is the problem of finding k seed nodes that maximize the scope of influence in a social network. Therefore, the comprehensive influence of node needs to be considered, when we choose the most influential node set consisted of k seed nodes. On account of the traditional methods used to measure the influence of nodes, such as degree centrality, betweenness centrality and closeness centrality, consider only a single aspect of the influence of node, so the influence measured by traditional methods mentioned above of node is not accurate. In this paper, we obtain the following result through experimental analysis: the influence of a node is relevant not only to its degree and coreness, but also to the degree and coreness of the n-order neighbor nodes. Hence, we propose a algorithm based on the mixed importance of nodes to measure the comprehensive influence of node, and the algorithm we proposed is simple and efficient. In addition, the performance of the algorithm we proposed is better than that of traditional influence maximization algorithms.Keywords
Cite This Article
Citations
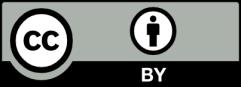