Open Access
ARTICLE
Fuzzy C-Means Algorithm Automatically Determining Optimal Number of Clusters
Air and Missile Defense College, Air Force Engineering University, Xi’an, 710051, China.
* Corresponding Author: Ruikang Xing. Email: .
Computers, Materials & Continua 2019, 60(2), 767-780. https://doi.org/10.32604/cmc.2019.04500
Abstract
In clustering analysis, the key to deciding clustering quality is to determine the optimal number of clusters. At present, most clustering algorithms need to give the number of clusters in advance for clustering analysis of the samples. How to gain the correct optimal number of clusters has been an important topic of clustering validation study. By studying and analyzing the FCM algorithm in this study, an accurate and efficient algorithm used to confirm the optimal number of clusters is proposed for the defects of traditional FCM algorithm. For time and clustering accuracy problems of FCM algorithm and relevant algorithms automatically determining the optimal number of clusters, kernel function, AP algorithm and new evaluation indexes were applied to improve the confirmation of complexity and search the scope of traditional fuzzy C-means algorithm, and evaluation of clustering results. Besides, three groups of contrast experiments were designed with different datasets for verification. The results showed that the improved algorithm improves time efficiency and accuracy to certain degree.Keywords
Cite This Article
Citations
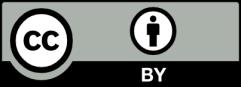
This work is licensed under a Creative Commons Attribution 4.0 International License , which permits unrestricted use, distribution, and reproduction in any medium, provided the original work is properly cited.