Open Access
ARTICLE
Retinal Vessel Extraction Framework Using Modified Adaboost Extreme Learning Machine
Department of Electronics and Communication Engineering, Velammal Institute of Technology, Chennai, 601204, Tamil Nadu, India.
Department of Information Technology, RMK Engineering College, Chennai, 601206, Tamil Nadu, India.
* Corresponding Author: B. V. Santhosh Krishna. Email: .
Computers, Materials & Continua 2019, 60(3), 855-869. https://doi.org/10.32604/cmc.2019.07585
Abstract
An explicit extraction of the retinal vessel is a standout amongst the most significant errands in the field of medical imaging to analyze both the ophthalmological infections, for example, Glaucoma, Diabetic Retinopathy (DR), Retinopathy of Prematurity (ROP), Age-Related Macular Degeneration (AMD) as well as non retinal sickness such as stroke, hypertension and cardiovascular diseases. The state of the retinal vasculature is a significant indicative element in the field of ophthalmology. Retinal vessel extraction in fundus imaging is a difficult task because of varying size vessels, moderately low distinction, and presence of pathologies such as hemorrhages, microaneurysms etc. Manual vessel extraction is a challenging task due to the complicated nature of the retinal vessel structure, which also needs strong skill set and training. In this paper, a supervised technique for blood vessel extraction in retinal images using Modified Adaboost Extreme Learning Machine (MAD-ELM) is proposed. Firstly, the fundus image preprocessing is done for contrast enhancement and in-homogeneity correction. Then, a set of core features is extracted, and the best features are selected using “minimal Redundancy-maximum Relevance (mRmR).” Later, using MAD-ELM method vessels and non vessels are classified. DRIVE and DR-HAGIS datasets are used for the evaluation of the proposed method. The algorithm’s performance is assessed based on accuracy, sensitivity and specificity. The proposed technique attains accuracy of 0.9619 on the DRIVE database and 0.9519 on DR-HAGIS database, which contains pathological images. Our results show that, in addition to healthy retinal images, the proposed method performs well in extracting blood vessels from pathological images and is therefore comparable with state of the art methods.Keywords
Cite This Article
B. V. Santhosh Krishna and T. Gnanasekaran, "Retinal vessel extraction framework using modified adaboost extreme learning machine," Computers, Materials & Continua, vol. 60, no.3, pp. 855–869, 2019. https://doi.org/10.32604/cmc.2019.07585Citations
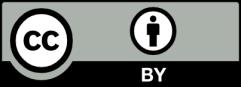