Open Access
ARTICLE
Multi-Label Learning Based on Transfer Learning and Label Correlation
College of Computer Science and Electronic Engineering and Key Laboratory for Embedded and Network Computing of Hunan Province, Hunan University, Changsha, 410082, China.
Oath Verizon Company, Manhattan, New York, 10007, USA.
* Corresponding Author: Kehua Yang. Email: .
Computers, Materials & Continua 2019, 61(1), 155-169. https://doi.org/10.32604/cmc.2019.05901
Abstract
In recent years, multi-label learning has received a lot of attention. However, most of the existing methods only consider global label correlation or local label correlation. In fact, on the one hand, both global and local label correlations can appear in real-world situation at same time. On the other hand, we should not be limited to pairwise labels while ignoring the high-order label correlation. In this paper, we propose a novel and effective method called GLLCBN for multi-label learning. Firstly, we obtain the global label correlation by exploiting label semantic similarity. Then, we analyze the pairwise labels in the label space of the data set to acquire the local correlation. Next, we build the original version of the label dependency model by global and local label correlations. After that, we use graph theory, probability theory and Bayesian networks to eliminate redundant dependency structure in the initial version model, so as to get the optimal label dependent model. Finally, we obtain the feature extraction model by adjusting the Inception V3 model of convolution neural network and combine it with the GLLCBN model to achieve the multi-label learning. The experimental results show that our proposed model has better performance than other multi-label learning methods in performance evaluating.Keywords
Cite This Article
Citations
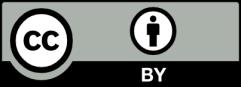