Open Access
ARTICLE
Keyphrase Generation Based on Self-Attention Mechanism
College of Computer Science and Electronic Engineering and Key Laboratory for Embedded and Network Computing of Hunan Province, Hunan University, Changsha, 410082, China.
Oath Verizon Company, Manhattan, New York, 10007, USA.
*Corresponding Author: Kehua Yang. Email: .
Computers, Materials & Continua 2019, 61(2), 569-581. https://doi.org/10.32604/cmc.2019.05952
Abstract
Keyphrase greatly provides summarized and valuable information. This information can help us not only understand text semantics, but also organize and retrieve text content effectively. The task of automatically generating it has received considerable attention in recent decades. From the previous studies, we can see many workable solutions for obtaining keyphrases. One method is to divide the content to be summarized into multiple blocks of text, then we rank and select the most important content. The disadvantage of this method is that it cannot identify keyphrase that does not include in the text, let alone get the real semantic meaning hidden in the text. Another approach uses recurrent neural networks to generate keyphrases from the semantic aspects of the text, but the inherently sequential nature precludes parallelization within training examples, and distances have limitations on context dependencies. Previous works have demonstrated the benefits of the self-attention mechanism, which can learn global text dependency features and can be parallelized. Inspired by the above observation, we propose a keyphrase generation model, which is based entirely on the self-attention mechanism. It is an encoder-decoder model that can make up the above disadvantage effectively. In addition, we also consider the semantic similarity between keyphrases, and add semantic similarity processing module into the model. This proposed model, which is demonstrated by empirical analysis on five datasets, can achieve competitive performance compared to baseline methods.Keywords
Cite This Article
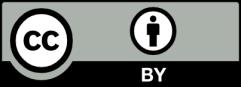