Open Access
ARTICLE
Unsupervised Anomaly Detection via DBSCAN for KPIs Jitters in Network Managements
1 College of Computer Science, National University of Defense Technology, Changsha, China.
2 School of Data Science and Computer Science, Sun Yat-sen University, Guangzhou, China.
3 College of Information and Engineering, Central South University, Changsha, China.
4 Faculty of Information Technology, Macau University of Science and Technology, Macau.
* Corresponding Author: Zhiping Cai. Email: .
Computers, Materials & Continua 2020, 62(2), 917-927. https://doi.org/10.32604/cmc.2020.05981
Abstract
For many Internet companies, a huge amount of KPIs (e.g., server CPU usage, network usage, business monitoring data) will be generated every day. How to closely monitor various KPIs, and then quickly and accurately detect anomalies in such huge data for troubleshooting and recovering business is a great challenge, especially for unlabeled data. The generated KPIs can be detected by supervised learning with labeled data, but the current problem is that most KPIs are unlabeled. That is a time-consuming and laborious work to label anomaly for company engineers. Build an unsupervised model to detect unlabeled data is an urgent need at present. In this paper, unsupervised learning DBSCAN combined with feature extraction of data has been used, and for some KPIs, its best F-Score can reach about 0.9, which is quite good for solving the current problem.Keywords
Cite This Article
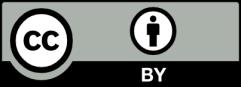
This work is licensed under a Creative Commons Attribution 4.0 International License , which permits unrestricted use, distribution, and reproduction in any medium, provided the original work is properly cited.