Open Access
ARTICLE
Defend Against Adversarial Samples by Using Perceptual Hash
1 Key Laboratory of Aerospace Information Security and Trusted Computing, Ministry of Education, School of Cyber Science and Engineering, Wuhan University, Wuhan, 430072, China.
2 School of Mathematics and Statistics, South Central University for Nationalities, Wuhan, 430074, China.
3 University of Central Florida, 4000 Central Florida Blvd. Orlando, Florida, 32816, USA.
* Corresponding Author: Dengpan Ye. Email: .
Computers, Materials & Continua 2020, 62(3), 1365-1386. https://doi.org/10.32604/cmc.2020.07421
Abstract
Image classifiers that based on Deep Neural Networks (DNNs) have been proved to be easily fooled by well-designed perturbations. Previous defense methods have the limitations of requiring expensive computation or reducing the accuracy of the image classifiers. In this paper, we propose a novel defense method which based on perceptual hash. Our main goal is to destroy the process of perturbations generation by comparing the similarities of images thus achieve the purpose of defense. To verify our idea, we defended against two main attack methods (a white-box attack and a black-box attack) in different DNN-based image classifiers and show that, after using our defense method, the attack-success-rate for all DNN-based image classifiers decreases significantly. More specifically, for the white-box attack, the attack-success-rate is reduced by an average of 36.3%. For the black-box attack, the average attack-successrate of targeted attack and non-targeted attack has been reduced by 72.8% and 76.7% respectively. The proposed method is a simple and effective defense method and provides a new way to defend against adversarial samples.Keywords
Cite This Article
Citations
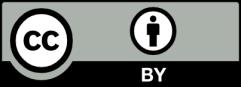