Open Access
ARTICLE
Human Action Recognition Based on Supervised Class-Specific Dictionary Learning with Deep Convolutional Neural Network Features
1 Key Laboratory of Advanced Process Control for Light Industry, Ministry of Education, Jiangnan University, Wuxi, China.
2 National Engineering Laboratory for Cereal Fermentation Technology, Jiangnan University, Wuxi, China.
* Corresponding Author: Binjie Gu. Email: .
Computers, Materials & Continua 2020, 63(1), 243-262. https://doi.org/10.32604/cmc.2020.06898
Received 09 April 2019; Accepted 13 June 2019; Issue published 30 March 2020
Abstract
Human action recognition under complex environment is a challenging work. Recently, sparse representation has achieved excellent results of dealing with human action recognition problem under different conditions. The main idea of sparse representation classification is to construct a general classification scheme where the training samples of each class can be considered as the dictionary to express the query class, and the minimal reconstruction error indicates its corresponding class. However, how to learn a discriminative dictionary is still a difficult work. In this work, we make two contributions. First, we build a new and robust human action recognition framework by combining one modified sparse classification model and deep convolutional neural network (CNN) features. Secondly, we construct a novel classification model which consists of the representation-constrained term and the coefficients incoherence term. Experimental results on benchmark datasets show that our modified model can obtain competitive results in comparison to other state-of-the-art models.Keywords
Cite This Article
Citations
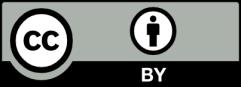