Open Access
ARTICLE
Feature Fusion Multi-View Hashing Based on Random Kernel Canonical Correlation Analysis
1 College of Computer Science and Information Technology, Central South University of Forestry & Technology, Changsha, 410114, China.
* Corresponding Authors: Jiaohua Qin. Email: ;
Computers, Materials & Continua 2020, 63(2), 675-689. https://doi.org/10.32604/cmc.2020.07730
Received 22 June 2019; Accepted 24 July 2019; Issue published 01 May 2020
Abstract
Hashing technology has the advantages of reducing data storage and improving the efficiency of the learning system, making it more and more widely used in image retrieval. Multi-view data describes image information more comprehensively than traditional methods using a single-view. How to use hashing to combine multi-view data for image retrieval is still a challenge. In this paper, a multi-view fusion hashing method based on RKCCA (Random Kernel Canonical Correlation Analysis) is proposed. In order to describe image content more accurately, we use deep learning dense convolutional network feature DenseNet to construct multi-view by combining GIST feature or BoW_SIFT (Bag-of-Words model+SIFT feature) feature. This algorithm uses RKCCA method to fuse multi-view features to construct association features and apply them to image retrieval. The algorithm generates binary hash code with minimal distortion error by designing quantization regularization terms. A large number of experiments on benchmark datasets show that this method is superior to other multi-view hashing methods.Keywords
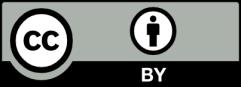