Open Access
ARTICLE
OTT Messages Modeling and Classification Based on Recurrent Neural Networks
1 School of Economics & Management, Beijing University of Posts and Telecommunications, Beijing, 100876, China.
2 School of Automation, Beijing University of Posts and Telecommunications, Beijing, 100876, China.
3 School of Electronic & Engineering, Beijing University of Posts and Telecommunications, Beijing, 100876, China.
4 Mehran University of Engineering & Technology, Jamshoro, Pakistan.
* Corresponding Author: Mengke Yang. Email: .
Computers, Materials & Continua 2020, 63(2), 769-785. https://doi.org/10.32604/cmc.2020.07528
Received 31 May 2019; Accepted 02 August 2019; Issue published 01 May 2020
Abstract
A vast amount of information has been produced in recent years, which brings a huge challenge to information management. The better usage of big data is of important theoretical and practical significance for effectively addressing and managing messages. In this paper, we propose a nine-rectangle-grid information model according to the information value and privacy, and then present information use policies based on the rough set theory. Recurrent neural networks were employed to classify OTT messages. The content of user interest is effectively incorporated into the classification process during the annotation of OTT messages, ending with a reliable trained classification model. Experimental results showed that the proposed method yielded an accurate classification performance and hence can be used for effective distribution and control of OTT messages.Keywords
Cite This Article
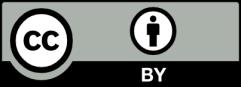