Open Access
ARTICLE
The Quantum Approximate Algorithm for Solving Traveling Salesman Problem
1 School of Computer Science and Technology, Anhui University of Technology, Maanshan, China.
2 School of Physics, University of Western Australia, Perth, Australia.
3 School of Computer Science and Engineering, Southeast University, Nanjing, China.
* Corresponding Authors: Yue Ruan. Email: ;
Jingbo Wang. Email: .
Computers, Materials & Continua 2020, 63(3), 1237-1247. https://doi.org/10.32604/cmc.2020.010001
Received 04 February 2020; Accepted 25 February 2020; Issue published 30 April 2020
Abstract
The Quantum Approximate Optimization Algorithm (QAOA) is an algorithmic framework for finding approximate solutions to combinatorial optimization problems. It consists of interleaved unitary transformations induced by two operators labelled the mixing and problem Hamiltonians. To fit this framework, one needs to transform the original problem into a suitable form and embed it into these two Hamiltonians. In this paper, for the well-known NP-hard Traveling Salesman Problem (TSP), we encode its constraints into the mixing Hamiltonian rather than the conventional approach of adding penalty terms to the problem Hamiltonian. Moreover, we map edges (routes) connecting each pair of cities to qubits, which decreases the search space significantly in comparison to other approaches. As a result, our method can achieve a higher probability for the shortest round-trip route with only half the number of qubits consumed compared to IBM Q’s approach. We argue the formalization approach presented in this paper would lead to a generalized framework for finding, in the context of QAOA, high-quality approximate solutions to NP optimization problems.Keywords
Cite This Article
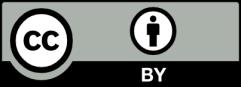