Open Access
ARTICLE
State-Based Control Feature Extraction for Effective Anomaly Detection in Process Industries
1 School of Information, Liaoning University, Shenyang, 110036, China.
2 School of Computer Science & Cyberspace Security, Hainan University, Haikou, 570228, China.
3 School of Informatics, Edinburgh University, Edinburgh, EH89AB, UK.
* Corresponding Author: Jiangyuan Yao. Email: .
Computers, Materials & Continua 2020, 63(3), 1415-1431. https://doi.org/10.32604/cmc.2020.09692
Received 15 January 2020; Accepted 28 February 2020; Issue published 30 April 2020
Abstract
In process industries, the characteristics of industrial activities focus on the integrality and continuity of production process, which can contribute to excavating the appropriate features for industrial anomaly detection. From this perspective, this paper proposes a novel state-based control feature extraction approach, which regards the finite control operations as different states. Furthermore, the procedure of state transition can adequately express the change of successive control operations, and the statistical information between different states can be used to calculate the feature values. Additionally, OCSVM (One Class Support Vector Machine) and BPNN (BP Neural Network), which are optimized by PSO (Particle Swarm Optimization) and GA (Genetic Algorithm) respectively, are introduced as alternative detection engines to match with our feature extraction approach. All experimental results clearly show that the proposed feature extraction approach can effectively coordinate with the optimized classification algorithms, and the optimized GA-BPNN classifier is suggested as a more applicable detection engine by comparing its average detection accuracies with the ones of PSOOCSVM classifier.Keywords
Cite This Article
Citations
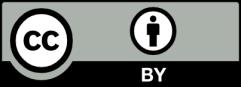