Open Access
ARTICLE
MII: A Novel Text Classification Model Combining Deep Active Learning with BERT
1 College of Computer Science and Technology, Nanjing University of Aeronautics and Astronautics,
Nanjing, 211106, China.
2 Key Laboratory of Safety-Critical Software, Ministry of Industry and Information Technology, Nanjing, 211106, China.
3 Collaborative Innovation Center of Novel Software Technology and Industrialization, Nanjing, 210046, China.
4 School of Information Technology and Electrical Engineering, University of Queensland, Queensland, Australia.
* Corresponding Author: Bohan Li. Email: .
Computers, Materials & Continua 2020, 63(3), 1499-1514. https://doi.org/10.32604/cmc.2020.09962
Received 31 January 2020; Accepted 01 March 2020; Issue published 30 April 2020
Abstract
Active learning has been widely utilized to reduce the labeling cost of supervised learning. By selecting specific instances to train the model, the performance of the model was improved within limited steps. However, rare work paid attention to the effectiveness of active learning on it. In this paper, we proposed a deep active learning model with bidirectional encoder representations from transformers (BERT) for text classification. BERT takes advantage of the self-attention mechanism to integrate contextual information, which is beneficial to accelerate the convergence of training. As for the process of active learning, we design an instance selection strategy based on posterior probabilities Margin, Intra-correlation and Inter-correlation (MII). Selected instances are characterized by small margin, low intra-cohesion and high inter-cohesion. We conduct extensive experiments and analytics with our methods. The effect of learner is compared while the effect of sampling strategy and text classification is assessed from three real datasets. The results show that our method outperforms the baselines in terms of accuracy.Keywords
Cite This Article
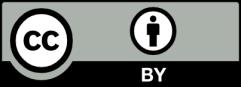