Open Access
ARTICLE
Machine Learning and Classical Forecasting Methods Based Decision Support Systems for COVID-19
1 Gumushane University, Bağlarbaşı Mah, Gümüşhane, 29000, Turkey.
2 Istanbul University-Cerrahpasa, İstanbul Üniversitesi Avcılar Kampüsü, İstanbul, 34320, Turkey.
* Corresponding Author: Ersin Namlı. Email: .
(This article belongs to the Special Issue: Artificial Intelligence and Information Technologies for COVID-19)
Computers, Materials & Continua 2020, 64(3), 1383-1399. https://doi.org/10.32604/cmc.2020.011335
Received 01 May 2020; Accepted 24 May 2020; Issue published 30 June 2020
Abstract
From late 2019 to the present day, the coronavirus outbreak tragically affected the whole world and killed tens of thousands of people. Many countries have taken very stringent measures to alleviate the effects of the coronavirus disease 2019 (COVID-19) and are still being implemented. In this study, various machine learning techniques are implemented to predict possible confirmed cases and mortality numbers for the future. According to these models, we have tried to shed light on the future in terms of possible measures to be taken or updating the current measures. Support Vector Machines (SVM), Holt-Winters, Prophet, and Long-Short Term Memory (LSTM) forecasting models are applied to the novel COVID-19 dataset. According to the results, the Prophet model gives the lowest Root Mean Squared Error (RMSE) score compared to the other three models. Besides, according to this model, a projection for the future COVID-19 predictions of Turkey has been drawn and aimed to shape the current measures against the coronavirus.Keywords
Cite This Article
R. Ünlü and E. Namlı, "Machine learning and classical forecasting methods based decision support systems for covid-19," Computers, Materials & Continua, vol. 64, no.3, pp. 1383–1399, 2020. https://doi.org/10.32604/cmc.2020.011335Citations
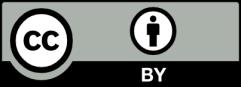