Open Access
ARTICLE
An Improved Method for the Fitting and Prediction of the Number of COVID-19 Confirmed Cases Based on LSTM
1 School of Computer Science and Cyberspace Security, Hainan University, Haikou, 570228, China.
2 College of Humanities and Communication, Hainan University, Haikou, 570228, China.
3 University of Chinese Academy of Science, Beijing, 100049, China.
4 National University of Defense Technology, Changsha, 410073, China.
5 Department of Mathematics and Computer Science, Northeastern State University, Tahlequah, USA.
* Corresponding Author: Xiangyan Tang. Email: .
(This article belongs to the Special Issue: Artificial Intelligence and Information Technologies for COVID-19)
Computers, Materials & Continua 2020, 64(3), 1473-1490. https://doi.org/10.32604/cmc.2020.011317
Received 30 April 2020; Accepted 29 May 2020; Issue published 30 June 2020
Abstract
New coronavirus disease (COVID-19) has constituted a global pandemic and has spread to most countries and regions in the world. Through understanding the development trend of confirmed cases in a region, the government can control the pandemic by using the corresponding policies. However, the common traditional mathematical differential equations and population prediction models have limitations for time series population prediction, and even have large estimation errors. To address this issue, we propose an improved method for predicting confirmed cases based on LSTM (Long-Short Term Memory) neural network. This work compares the deviation between the experimental results of the improved LSTM prediction model and the digital prediction models (such as Logistic and Hill equations) with the real data as reference. Furthermore, this work uses the goodness of fitting to evaluate the fitting effect of the improvement. Experiments show that the proposed approach has a smaller prediction deviation and a better fitting effect. Compared with the previous forecasting methods, the contributions of our proposed improvement methods are mainly in the following aspects: 1) we have fully considered the spatiotemporal characteristics of the data, rather than single standardized data. 2) the improved parameter settings and evaluation indicators are more accurate for fitting and forecasting. 3) we consider the impact of the epidemic stage and conduct reasonable data processing for different stage.Keywords
Cite This Article
Citations
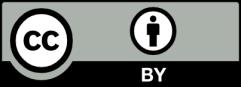