Open Access
ARTICLE
Better Visual Image Super-Resolution with Laplacian Pyramid of Generative Adversarial Networks
1 School of Computer Science and Engineering, Central South University, Changsha, 410000, China.
2 Apple Inc., California, 95014, USA.
* Corresponding Author: Xin Yao. Email: .
Computers, Materials & Continua 2020, 64(3), 1601-1614. https://doi.org/10.32604/cmc.2020.09754
Received 17 January 2020; Accepted 03 March 2020; Issue published 30 June 2020
Abstract
Although there has been a great breakthrough in the accuracy and speed of super-resolution (SR) reconstruction of a single image by using a convolutional neural network, an important problem remains unresolved: how to restore finer texture details during image super-resolution reconstruction? This paper proposes an Enhanced Laplacian Pyramid Generative Adversarial Network (ELSRGAN), based on the Laplacian pyramid to capture the high-frequency details of the image. By combining Laplacian pyramids and generative adversarial networks, progressive reconstruction of super-resolution images can be made, making model applications more flexible. In order to solve the problem of gradient disappearance, we introduce the Residual-in-Residual Dense Block (RRDB) as the basic network unit. Network capacity benefits more from dense connections, is able to capture more visual features with better reconstruction effects, and removes BN layers to increase calculation speed and reduce calculation complexity. In addition, a loss of content driven by perceived similarity is used instead of content loss driven by spatial similarity, thereby enhancing the visual effect of the superresolution image, making it more consistent with human visual perception. Extensive qualitative and quantitative evaluation of the baseline datasets shows that the proposed algorithm has higher mean-sort-score (MSS) than any state-of-the-art method and has better visual perception.Keywords
Cite This Article
Citations
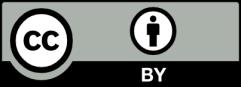