Open Access
ARTICLE
Using Object Detection Network for Malware Detection and Identification in Network Traffic Packets
1 School of Information Science and Technology, North China University of Technology, Beijing, 100144, China.
2 Department of Computer Science, University of Illinois Springfield, Springfield, USA.
* Corresponding Author: Yanhui Guo. Email: .
Computers, Materials & Continua 2020, 64(3), 1785-1796. https://doi.org/10.32604/cmc.2020.010091
Received 10 February 2020; Accepted 24 April 2020; Issue published 30 June 2020
Abstract
In recent years, the number of exposed vulnerabilities has grown rapidly and more and more attacks occurred to intrude on the target computers using these vulnerabilities such as different malware. Malware detection has attracted more attention and still faces severe challenges. As malware detection based traditional machine learning relies on exports’ experience to design efficient features to distinguish different malware, it causes bottleneck on feature engineer and is also time-consuming to find efficient features. Due to its promising ability in automatically proposing and selecting significant features, deep learning has gradually become a research hotspot. In this paper, aiming to detect the malicious payload and identify their categories with high accuracy, we proposed a packet-based malicious payload detection and identification algorithm based on object detection deep learning network. A dataset of malicious payload on code execution vulnerability has been constructed under the Metasploit framework and used to evaluate the performance of the proposed malware detection and identification algorithm. The experimental results demonstrated that the proposed object detection network can efficiently find and identify malicious payloads with high accuracy.Keywords
Cite This Article
Citations
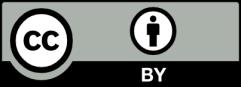