Open Access
ARTICLE
A Recommendation Method for Highly Sparse Dataset Based on Teaching Recommendation Factorization Machines
1 School of Computer Science and Engineering, Huaihua University, Huaihua, 418000, China.
2 Key Laboratory of Wuling-Mountain Health Big Data Intelligent Processing and Application in Hunan
Province Universities, Huaihua, 418000, China.
3 Key Laboratory of Intelligent Control Technology for Wuling-Mountain Ecological Agriculture in Hunan
Province, Huaihua, 418000, China.
4 School of Computer, Wuhan University, Wuhan, 430072, China.
5 Department of Electrical and Computer Engineering, Duke University, Durham, NC 27708, USA.
6 School of Computer Science and Engineering, Yulin Normal University, Yulin, 537000, China.
* Corresponding Author: Shijun Li. Email: .
Computers, Materials & Continua 2020, 64(3), 1959-1975. https://doi.org/10.32604/cmc.2020.010186
Received 15 February 2020; Accepted 03 May 2020; Issue published 30 June 2020
Abstract
There is no reasonable scientific basis for selecting the excellent teachers of the school’s courses. To solve the practical problem, we firstly give a series of normalization models for defining the key attributes of teachers’ professional foundation, course difficulty coefficient, and comprehensive evaluation of teaching. Then, we define a partial weight function to calculate the key attributes, and obtain the partial recommendation values. Next, we construct a highly sparse Teaching Recommendation Factorization Machines (TRFMs) model, which takes the 5-tuples relation including teacher, course, teachers’ professional foundation, course difficulty, teaching evaluation as the feature vector, and take partial recommendation value as the recommendation label. Finally, we design a novel Top-N excellent teacher recommendation algorithm based on TRFMs by course classification on the highly sparse dataset. Experimental results show that the proposed TRFMs and recommendation algorithm can accurately realize the recommendation of excellent teachers on a highly sparse historical teaching dataset. The recommendation accuracy is superior to that of the three-dimensional tensor decomposition model algorithm which also solves sparse datasets. The proposed method can be used as a new recommendation method applied to the teaching arrangements in all kinds of schools, which can effectively improve the teaching quality.Keywords
Cite This Article
Citations
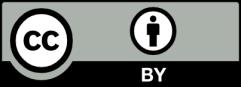
This work is licensed under a Creative Commons Attribution 4.0 International License , which permits unrestricted use, distribution, and reproduction in any medium, provided the original work is properly cited.