Open Access
ARTICLE
A Novel Method of Heart Failure Prediction Based on DPCNNXGBOOST Model
1 Chengdu Institute of Computer Applications, Chinese Academy of Sciences, Chengdu, 610041, China.
2 Chongqing Institute of Green and Intelligent Technology, Chinese Academy of Sciences, Chongqing, 400714, China.
3 School of Computer and Control Engineering, University of Chinese Academy of Sciences, Beijing, 100049, China.
4 Department of Anesthesia, Southwest Hospital, Army Medical University, Chongqing, 400038, China.
* Corresponding Author: Yuwen Chen. Email: .
Computers, Materials & Continua 2020, 65(1), 495-510. https://doi.org/10.32604/cmc.2020.011278
Received 29 April 2020; Accepted 21 May 2020; Issue published 23 July 2020
Abstract
The occurrence of perioperative heart failure will affect the quality of medical services and threaten the safety of patients. Existing methods depend on the judgment of doctors, the results are affected by many factors such as doctors’ knowledge and experience. The accuracy is difficult to guarantee and has a serious lag. In this paper, a mixture prediction model is proposed for perioperative adverse events of heart failure, which combined with the advantages of the Deep Pyramid Convolutional Neural Networks (DPCNN) and Extreme Gradient Boosting (XGBOOST). The DPCNN was used to automatically extract features from patient’s diagnostic texts, and the text features were integrated with the preoperative examination and intraoperative monitoring values of patients, then the XGBOOST algorithm was used to construct the prediction model of heart failure. An experimental comparison was conducted on the model based on the data of patients with heart failure in southwest hospital from 2014 to 2018. The results showed that the DPCNN-XGBOOST model improved the predictive sensitivity of the model by 3% and 31% compared with the text-based DPCNN Model and the numeric-based XGBOOST Model.Keywords
Cite This Article
Citations
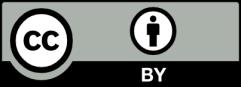