Open Access
ARTICLE
IWD-Miner: A Novel Metaheuristic Algorithm for Medical Data Classification
Department of Computer Science, College of Computer and Information Sciences, King Saud University, Riyadh, 11362, Saudi Arabia
* Corresponding Author: Sarab AlMuhaideb. Email:
(This article belongs to the Special Issue: AI, IoT, Blockchain Assisted Intelligent Solutions to Medical and Healthcare Systems)
Computers, Materials & Continua 2021, 66(2), 1329-1346. https://doi.org/10.32604/cmc.2020.013576
Received 11 August 2020; Accepted 19 September 2020; Issue published 26 November 2020
Abstract
Medical data classification (MDC) refers to the application of classification methods on medical datasets. This work focuses on applying a classification task to medical datasets related to specific diseases in order to predict the associated diagnosis or prognosis. To gain experts’ trust, the prediction and the reasoning behind it are equally important. Accordingly, we confine our research to learn rule-based models because they are transparent and comprehensible. One approach to MDC involves the use of metaheuristic (MH) algorithms. Here we report on the development and testing of a novel MH algorithm: IWD-Miner. This algorithm can be viewed as a fusion of Intelligent Water Drops (IWDs) and AntMiner+. It was subjected to a four-stage sensitivity analysis to optimize its performance. For this purpose, 21 publicly available medical datasets were used from the Machine Learning Repository at the University of California Irvine. Interestingly, there were only limited differences in performance between IWD-Miner variants which is suggestive of its robustness. Finally, using the same 21 datasets, we compared the performance of the optimized IWD-Miner against two extant algorithms, AntMiner+ and J48. The experiments showed that both rival algorithms are considered comparable in the effectiveness to IWD-Miner, as confirmed by the Wilcoxon nonparametric statistical test. Results suggest that IWD-Miner is more efficient than AntMiner+ as measured by the average number of fitness evaluations to a solution (1,386,621.30 vs. 2,827,283.88 fitness evaluations, respectively). J48 exhibited higher accuracy on average than IWD-Miner (79.58 vs. 73.65, respectively) but produced larger models (32.82 leaves vs. 8.38 terms, respectively).Keywords
Cite This Article
S. AlMuhaideb, R. BinGhannam, N. Alhelal, S. Alduheshi, F. Alkhamees et al., "Iwd-miner: a novel metaheuristic algorithm for medical data classification," Computers, Materials & Continua, vol. 66, no.2, pp. 1329–1346, 2021. https://doi.org/10.32604/cmc.2020.013576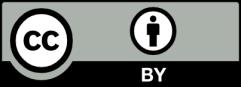