Open Access
ARTICLE
Estimating Loss Given Default Based on Beta Regression
1 Department of Risk Management and Insurance, The University of Jordan, Aqaba, 77111, Jordan
2 Universiti Kebangsaan Malaysia, Department of Mathematical Sciences, Malaysia
3 Department of Basic Sciences, College of Science and Theoretical Studies, Saudi Electronic University, Riyadh, 11673, Saudi Arabia
* Corresponding Author: Nawaf N. Hamadneh. Email:
Computers, Materials & Continua 2021, 66(3), 3329-3344. https://doi.org/10.32604/cmc.2021.014509
Received 24 September 2020; Accepted 19 October 2020; Issue published 28 December 2020
Abstract
Loss given default (LGD) is a key parameter in credit risk management to calculate the required regulatory minimum capital. The internal ratings-based (IRB) approach under the Basel II allows institutions to determine the loss given default (LGD) on their own. In this study, we have estimated LGD for a credit portfolio data by using beta regression with precision parameter (∅) and mean parameter (μ). The credit portfolio data was obtained from a banking institution in Jordan; for the period of January 2010 until December 2014. In the first stage, we have used the “outstanding amount” and “amount of borrowing” to find LGD of each default borrower (494 out of 4393 borrower). In the second stage, we fit univariate parametric distributions to the LGD data to obtain the beta distribution. After that, we have estimated the values of ∅ based on microeconomic variables (SPP, OE and LR). Moreover, we have estimated the values of μ based on macroeconomic variables (GDP and Inflation rate). Finally, we have compared between six different link functions (Logit, loglog, probit, cloglog, cauchit, and log), which have used with ∅ and μ. The results show that Beta regression with probit link function has the highest R-squared with accepted measurements for logL, AIC and BIC.Keywords
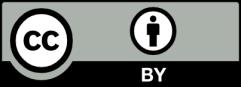